Lin Chen, PhD
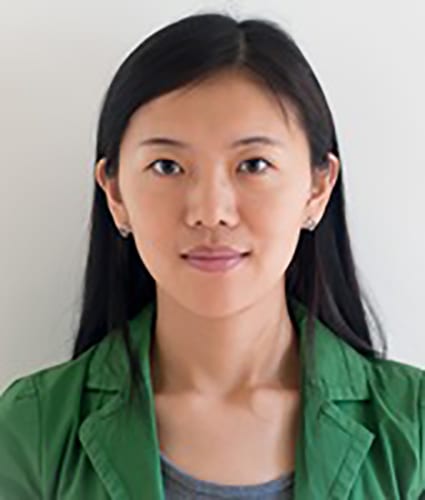
Lin Chen’s overall research interests focus on the development of statistical methods for analyzing high-dimensional genomics data. Her methodology developments are always motivated by challenges in real data problems. She develops methods to analyze high-dimensional ‘omics’ data from genetic association studies, next-generation sequencing studies, gene transcriptional expression studies, proteomic studies and more recently, the integration of ‘big data’.
These are some of her in depth research interests:
- Multivariate analysis in genetic association studies
In the post-genomic era, over thousands of genome-wide association (GWA) studies and state-of-the-art next-generation sequencing studies have been conducted on various human traits/diseases, producing a tremendous rich source of data. She focuses on developing methods to jointly analyze functionally related biological information and/or integrate a priori knowledge in association tests to identify genetic risk factors. Her group has developed methods for analyzing gene-sets/pathways, gene-based or set-based association testing methods, methods for detecting gene-environment interactions and gene-gene interactions, and they evaluated the effects of population stratification in set-based association tests. This part of work is partially supported by NIH R03CA174984, PI: Chen, Lin (2013-2015).
- Methods for complex missing data in proteomics studies
In the post-genomic era, over thousands of genome-wide association (GWA) studies and state-of-the-art next-generation sequencing studies have been conducted on various human traits/diseases, producing a tremendous rich source of data. She focuses on developing methods to jointly analyze functionally related biological information and/or integrate a priori knowledge in association tests to identify genetic risk factors. Her group has developed methods for analyzing gene-sets/pathways, gene-based or set-based association testing methods, methods for detecting gene-environment interactions and gene-gene interactions, and they evaluated the effects of population stratification in set-based association tests. This part of work is partially supported by NIH R03CA174984, PI: Chen, Lin (2013-2015).
- Methods for integrative genomics
Despite the promising progress made in recent human eQTL studies, genome-wide identification of trans-eQTLs is still a daunting task. The challenges stem not only from the high data-dimensionality, but also from the complex multiple-to-multiple relationships — one genetic variant may be associated to multiple expressions and one expression may be associated to multiple variants. A system-level analysis could better reveal the underlying biological mechanism and disease etiology. Lin’s group is interested in developing novel statistical tools (1) to construct eQTL networks. Such networks can reveal higher level structures beyond marginal associations; (2) to jointly model eQTL networks together with (undirected) gene expression networks; (3) to characterize the changes of eQTL regulatory patterns across different environments or phenotypes. This part of work is partially supported by R01GM108711, PI: Chen, Lin (2014-2019). In order to synthesize new knowledge about the organization of gene expression across human tissues, the Genotype-Tissue Expression (GTEx) project collected the transcriptome data from a wide variety of tissues from a large numbers of individuals. However, some human tissues are hardly accessible and are missing in the GTEx data. Our group is working on developing methods to impute transcriptome data in inaccessible tissues. Those results would not only benefit future studies on gene expression, but also provide insights into inter-tissue relatedness and the regulatory roles of cis- and trans-eQTLs in different tissues. Furthermore, by leveraging the inter-tissue predictability and the accessibility of tissues, Lin’s group suggests cost-effective strategies for collecting multi-tissue expression data in future projects. This work is supported by R01 MH101820, PI: Cox, Nancy. (2013-2016). In addition to her statistical methodology work, She is committed to develop computational tools for the proposed methods. She has released the following R software packages: ‘Trigger’ for integrative genomic and eQTL analysis, ‘SNPath’ for pathway analysis in association studies, ‘RHT’ for pathway analysis in proteomics studies, ‘EigenR2’ for dissecting variation in high-dimensional data, and `PEMM’ for abundance-dependent incomplete data analysis with proteomics data. Those are available through CRAN or Bioconductor.
- Ph.D. in Biostatistics, 2008, University of Washington, Seattle Advisor: Dr. John D. Storey
- B.S. in Economics, 2002, Peking University, Beijing, China
- Chen LS, Prentice RL and Wang P. (2014) A penalized EM algorithm for multivariate Gaussian data with non-ignorable missing data. Biometrics. 70(2):312-22
- Liu Q, Nicolae DL* and Chen LS*. (2013) Marbled Inflation from Population Structure in Gene-based Association Studies with Rare Variants. Genetic Epidemiology. 37(3): 286-292.* Joint correspondence
- Chen LS, Hsu L, Gamazon ER, Cox NJ, Nicolae DL. (2012) An exponential combination procedure for set-based association tests in sequencing studies. American Journal of Human Genetics. 91(6):977-986.
- Chen LS, Hsu L, Gamazon ER, Cox NJ, Nicolae DL. (2012) An exponential combination procedure for set-based association tests in sequencing studies. American Journal of Human Genetics. In press.
- Hutter CM, Chang-Claude J, Slattery ML, Pflugeisen BM, Lin Y, Duggan D, Nan H, Lemire M, Rangrej J, Figueiredo JC, Jiao S, Harrison TA, Liu Y, Chen LS, Stelling DL, Warnick GS, Hoffmeister M, Küry S, Fuchs CS, Giovannucci E, Hazra A, Kraft P, Hunter DJ, Gallinger S, Zanke BW, Brenner H, Frank B, Ma J, Ulrich CM, White E, Newcomb PA, Kooperberg C, LaCroix AZ, Prentice RL, Jackson RD, Schoen RE, Chanock SJ, Berndt SI, Hayes RB, Caan BJ, Potter JD, Hsu L, Bézieau S, Chan AT, Hudson TJ, Peters U. (2012) Characterization of gene-environment interactions for colorectal cancer susceptibility loci. Cancer Research, 72(8):2036-2044.
- Chen LS. (2012) Using eQTLs to reconstruct gene regulatory networks. Quantitative Trait Loci (QTL), 871:175-189
- Peters U, Hutter CM, Hsu L, Schumacher FR, Conti DV, Carlson CS, Edlund CK, Haile RW, Gallinger S, Zanke BW, Lemire M, Rangrej J, Vijayaraghavan R, Chan AT, Hazra A, Hunter DJ, Ma J, Fuchs CS, Giovannucci EL, Kraft P, Liu Y, Chen L, Jiao S, Makar KW, Taverna D, Gruber SB, Rennert G, Moreno V, Ulrich CM, Woods MO, Green RC, Parfrey PS, Prentice RL, Kooperberg C, Jackson RD, Lacroix AZ, Caan BJ, Hayes RB, Berndt SI, Chanock SJ, Schoen RE, Chang-Claude J, Hoffmeister M, Brenner H, Frank B, Bézieau S, Küry S, Slattery ML, Hopper JL, Jenkins MA, Le Marchand L, Lindor NM, Newcomb PA, Seminara D, Hudson TJ, Duggan DJ, Potter JD, Casey G. (2012) Meta-analysis of new genome-wide association studies of colorectal cancer risk. Hum Genet., 131:217-234.
- Chen LS, Debashis Paul, Prentice RL and Wang P. (2011) A regularized Hotelling’s T2 test for pathway analysis in proteomics studies. Journal of the American Statistical Association, 106(496): 1345-1360.
- Prentice RL, Paczesny S, Aragaki A, Amon L, Chen LS, Pitteri S, McIntosh M, Wang P, Hsia J, Jackson R, Rossouw JE, Manson JE, Johnson K, Eaton C, Hanash SM. (2010) Plasma protein concentrations and the risk of coronary heart disease and stroke among postmenopausal women. Genome Medicine. 2:48.
- Chen LS, Hutter CM, Potter JD, Liu Y, Prentice RL, Peters U and Hsu L. (2010) Insights into colon cancer etiology using a regularized approach to gene set analysis of GWAS data. American Journal of Human Genetics. 86 (6): 860-871.
- Pitteri SJ, Hanash SM, Agragaki A, Amon L, Chen LS, Buson TB, Paczesny S, Katayama H, Wang H, Johnson MM, Zhang Q, McIntosh M, Wang P, Kooperberg C, Rossouw JE, Jackson R, Manson JE, Hsia J, Liu S, Martin L and Prentice RL. (2009) Postmenopausal estrogen and progestin effects on the serum proteome. Genome Medicine, 1(12): 121.
- Chen LS and Storey JD. (2008) Eigen-R2 for dissecting the variation of high-dimensional studies, Bioinformatics, 24 (19): 2260-2262.
- Chen LS, Emmert-Streib F, and Storey JD. (2007) Harnessing naturally randomized transcription to infer regulatory relationships among genes. Genome Biology, 8: R219.
- Chen LS and Storey JD. (2006) Relaxed significance criteria for linkage analysis. Genetics, 173: 2371-2381.
- Applied Regression Analysis, University of Chicago, 2011, 2012, 2013, 2016, 2017
- Introduction to Biostatistics, University of Chicago, 2011, 2012, 2014
- Statistical Analysis with Missing Data, University of Chicago, 2016
- Introductory Statistical Genetics, University of Chicago, 2015 (with Novembre J and Pierce BL)
Software
- GMAC: Genomic Mediation Analysis with Adaptive Confounding Adjustment. [CRAN]
- Primo: Package in R for Integrative Multi-Omics analysis. [GitHub]
- mvMISE: Multivariate mixed-effects selection models. [GitHub]
- ofGEM: A test for omnibus-filtering-based gene-environment interactions meta-analysis. [GitHub]
- MixRF: A random-forest approach for imputing clustered incomplete data. [CRAN][GitHub]
- mixEMM: A mixed-effects model for analyzing cluster-level non-ignorable missing data.[CRAN]
- PEMM: A penalized EM algorithm for analyzing abundance-dependent incomplete data in proteomics studies. [CRAN]
- Trigger: Integrative genomic analysis. [Bioconductor]
- RHT: Pathway analysis in omics data. [CRAN]
- SNPath: Pathway analysis in association study. [GitHub] [Tutorial]
- EigenR2: For dissecting variation in high-dimensional data. [GitHub]